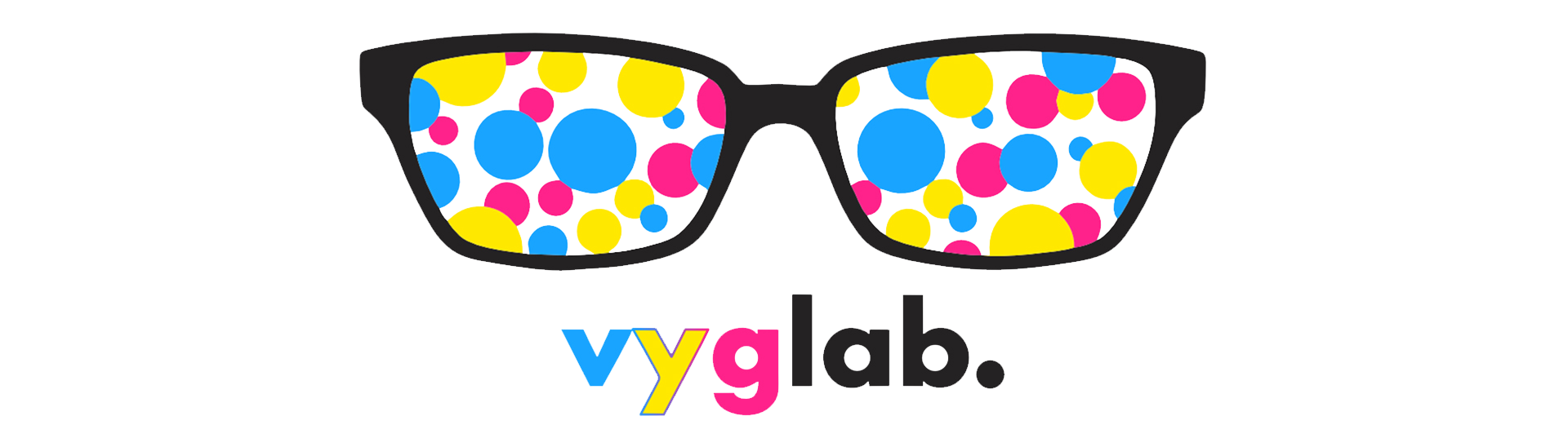
Depth Estimation and Semantic Segmentation
Today, the increasing availability of cameras makes image capture a daily reality, constantly accelerating the amount of visual data. The understanding of the image content, however, is a complex task generally associated with human intelligence.
When we take a picture, a three-dimensional scene is projected to a plane. The image can be seen as a simplification of a complex scene to an array of values representing color information at each position, known as pixels. Automatically understanding the scene translates into designing algorithms that are capable of obtaining high-level information from this array of values. However, handling the enormous number of variations that could be present in an image turns to be impossible in practice. Thus, tackling the problem as a data-driven problem seems the only viable solution.
In the last years, advances in Deep Learning, along with the increasing availability of data, led Scene Understanding to stop being a distant goal and turn into a reality. Understanding the geometry of a scene, that is, knowing the distance from the camera to the objects, and recognition of the objects present in the scene are two main objectives in Computer Vision, known as Depth Estimation and Semantic Segmentation, respectively.
At the VyGLab we are working on both topics, searching for new techniques and improvements at the frontiers of Deep Learning, experimenting with datasets from current problematics, as urban images for the development of autonomous cars.
People Involved in this Topic...
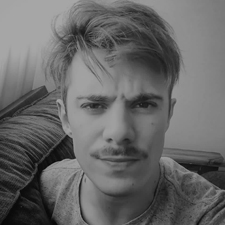
Ing. Juan I. Larregui
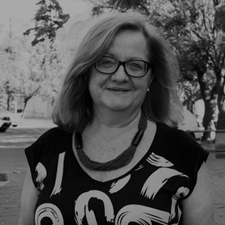
Dra. Silvia M. Castro